
Maria Chojnowska
15 May 2023, 4 min read
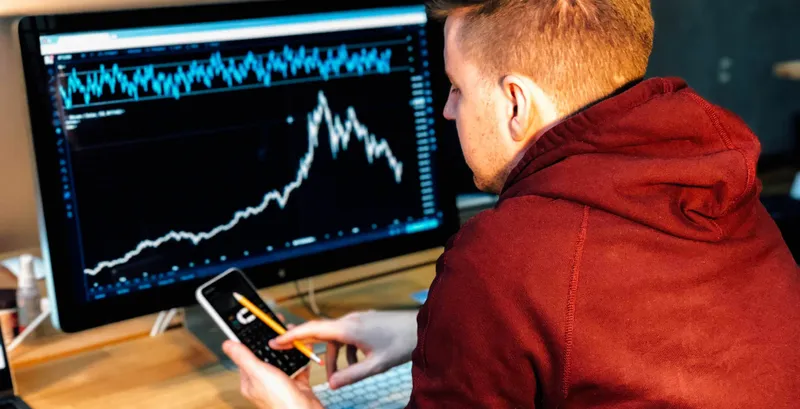
What's inside
Probably most of you have heard much about Data Engineering in recent years. It’s a topic that is growing more and more popular these days. This article will look at the most popular use cases of Data Engineering in the Finance Sector.
What is Data Engineering?
So before we jump into the specifics, let’s quickly remind ourselves what data engineering is. Simply put, it is the process of designing, building, and maintaining the data infrastructure necessary to support collecting, processing, storing, and analyzing large amounts of data.
It enables organizations to make sense of their data and turn it into valuable insights that inform business decisions. As you can imagine, no wonder that data engineering has become a vital component of the finance industry that creates and processes large amounts of data.
So, what are the most popular use cases?
Compliance
Most of you probably know how regulated the finance industry is. There are numerous regulations, and ensuring compliance with them can be time-consuming.
This is where data engineering can help save a lot of precious resources by streamlining the process. To do that, data engineering teams build data pipelines that aggregate data from multiple sources, making it much easier to run compliance checks.
These pipelines must be designed to capture all relevant data and metadata, ensuring compliance teams can access the information they need to demonstrate regulatory compliance. Data engineering teams must also ensure the pipelines are secure, reliable, and auditable. All of this allows us to maintain the trust of regulators and stakeholders.
Risk Management
Another important part of the fintech landscape where data engineering is applied, Financial institutions use risk models to make investment decisions, evaluate loans and other financial products, and manage portfolio risk. These models require large amounts of historical data and complex analytics, making data engineering essential to building and maintaining them.
Data engineering departments develop and manage the data infrastructure necessary to support these models (like pipelines, data warehouses, or data lakes). They must ensure that the data is accurate, reliable, and up-to-date. It must also be accessed and processed efficiently by risk analysts.
Fraud Detection
Data engineering is essential in the field of fraud detection. Financial organizations use complex machine learning models to recognize and flag suspicious, and possibly fraudulent transactions. Data engineering is a crucial facilitator since these models need high-throughput data pipelines that process enormous volumes of data in real time.
Data engineering teams develop and deploy these fraud detection systems, which ensure they can handle data from many sources, including internal transaction data, third-party data, and social media feeds.
For these systems to continue to trust customers and authorities, they must be highly scalable, fault-tolerant, and secure.
Trading
Trading based on algorithms has become increasingly popular in the finance industry. One can only imagine that a computer (given the sufficient amount of historical data) can help to make trading decisions faster than any human. There are cases where the algorithm can do the trading itself. It’s probably obvious that machine learning models are used to make those trading decisions. Data engineering teams are critical in building the pipelines that feed data into these models.
Those data pipelines must be highly scalable and able to process enormous amounts of data in real-time, ensuring that the trading models can access the most recent data. Data engineering teams must also ensure that the systems that execute trades are reliable, performant, and secure, which minimizes the risk of errors or fraud.
Customer Analytics
Customer analysis is where data engineering tends to be applied. It’s an essential aspect of any fintech product as it allows us to gain and analyze insights into customer behavior. This will enable them to drastically improve customer experience and keep retention at the desired level. Financial institutions can collect enormous customer data, like demographic and economic information, transaction history, and even social media activity.
Summary
As you can see, data engineering plays a significant role in fintech products as they collect large amounts of data and can use this information to make their products better and the lives of their customers easier.
If you own or want to build a fintech product and want to know how data engineering can improve your project, do not hesitate to drop us a line. At Sunscrapers, we have all the necessary knowledge and experience to help you and are excited to introduce this trend.