
Przemek Lewandowski
1 March 2023, 5 min read
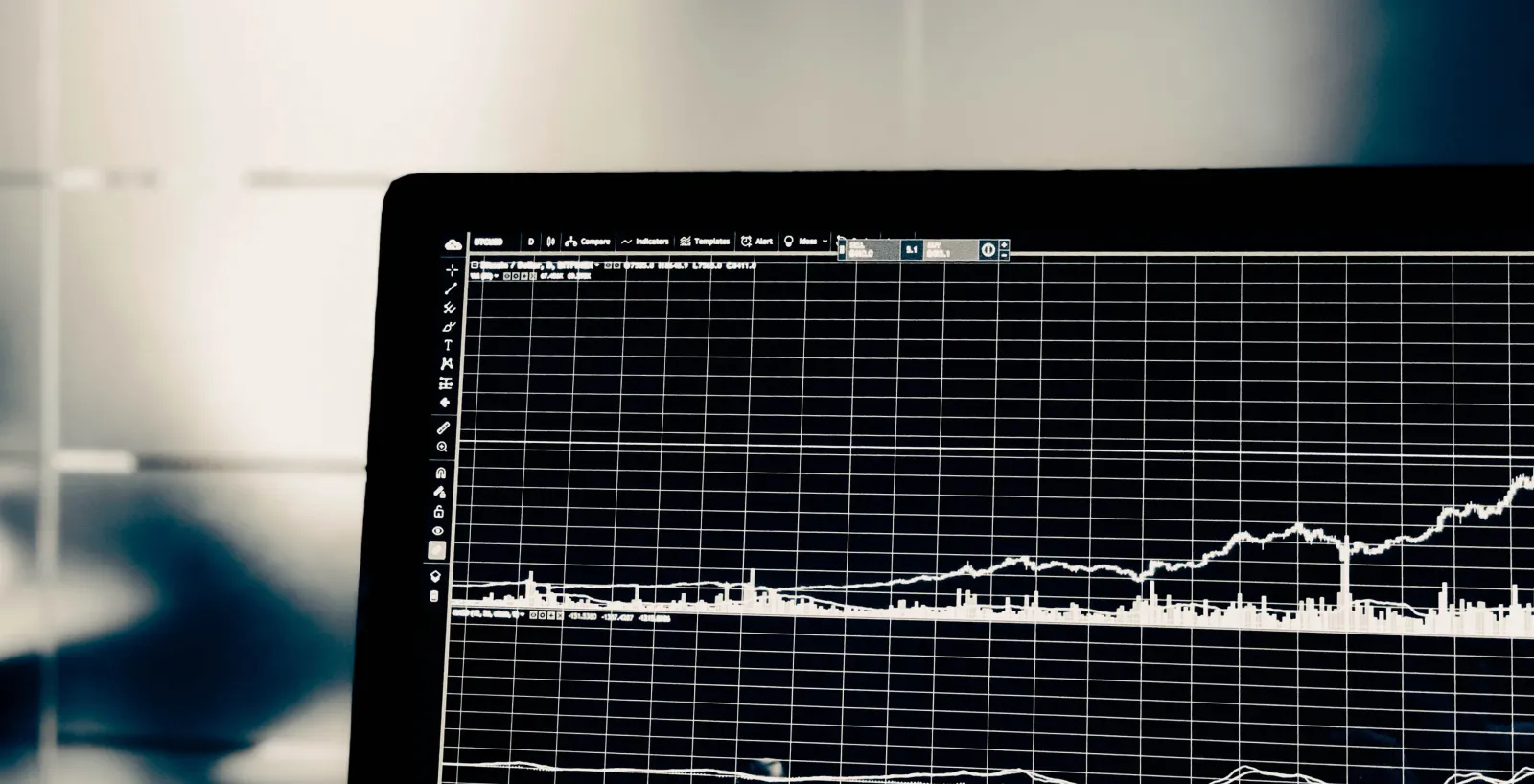
What's inside
- What is data cleaning, and why should you care?
- What are the steps of data cleaning?
- Here’s why data cleaning is essential
- Cleaning your data is a must
Small businesses can get away with a few Excel spreadsheets for tracking their operations. However, as these companies continue to grow, they can no longer keep up the speed using this simple method. At one point or another, data begins to pour in, and a single-page spreadsheet transforms into a database that later grows into a data warehouse.
Without proper investment in data science projects, these companies will never unlock the potential of this data to accelerate their growth and increase their operational efficiency (for example, in building better products or delivering better services).
Organizations that want to win in their markets need to know where to find the data they need and how it all ties together. But before setting out to analyze data, they need to ensure that their data sets are clean.
Innovative companies are aware of the importance of data cleaning.
Read on to find out what data clean cleaning is and why it is crucial for analytics and business intelligence.
What's inside
01 What is data cleaning, and why should you care? 02 What are the steps of data cleaning? 03 Here’s why data cleaning is essential 04 Cleaning your data is a must
What is data cleaning, and why should you care?
Datasets usually contain large volumes of data that may be stored in formats that are not easy to use. That’s why data scientists need first to make sure that data is correctly formatted and conforms to the set of rules.
Moreover, combining data from different sources can be tricky, and another job of data scientists is making sure that the resulting combination of information makes sense.
Data sparseness and formatting inconsistencies are the biggest challenges – and that’s what data cleansing is all about. Data cleaning is a task that identifies incorrect, incomplete, inaccurate, or irrelevant data, fixes the problems, and ensures that all such issues will be resolved automatically.
What are the steps of data cleaning?
Some of the most common steps and methods of data cleansing experienced development teams swear by
- Dealing with missing data
- Standardizing the process
- Validating data accuracy
- Removing duplicate data
- Handling structural errors
- Getting rid of unwanted observations
Let's delve into the details of three selected methods:
- Dealing with missing data - ignoring missing values in a data set is a huge mistake as most algorithms simply don't accept them. Some companies deal with this problem by imputing the missing values based on other observations or dropping the observations with missing values altogether. But these strategies lead to loss of information (note that "no value" also tells us something. Companies that miss categorical data can label them as "Missing." Missing numeric data should be flagged and filled with 0 to allow the algorithm to estimate the optimal constant for such a situation.
- Structural errors - these are mistakes that arise during measurement, transferring data, and other issues that emerge out of poor data management. Inconsistent punctuation, typos, and mislabeled classes are the most common problems. Such errors illustrate data cleaning importance really well.
- Unwanted observations - companies dealing with data science often encounter unwanted observations in the data sets. These can be duplicate observations or irrelevant ones for the specific problem they're trying to solve. Checking for irrelevant observations is a great strategy for streamlining the process of engineering features - the development team will have a much easier time building model.
Want to learn more? Read this article for a detailed overview and code samples: Quick guide to data cleaning with examples.
Here’s why data cleaning is essential
Data quality is of central importance to enterprises that rely on data for maintaining their operations. To give you an example, businesses need to make sure that accurate invoices are emailed to the right customers. Companies must focus on data quality to make the most of customer data and boost the brand's value.
Here are some more benefits data cleansing brings to enterprises.
- Avoid costly errors
Data cleansing is the best solution for clearing the costs that crop up when organizations are busy processing errors, correcting incorrect data, or troubleshooting.
- Boost customer acquisition
Organizations that maintain their databases in shape can develop lists of prospects using accurate and updated data. As a result, they increase the efficiency of their customer acquisition and reduce its cost.
- Make sense of data across different channels
Data cleaning clears the way to managing multichannel customer data seamlessly, allowing organizations to find opportunities for successful marketing campaigns and new methods for reaching their target audiences.
- Improve the decision-making process
Nothing helps to boost a decision-making process like clean data. Accurate and updated data supports analytics and business intelligence that, in turn, provide organizations with resources for better decision-making and execution.
- Increase employee productivity
Clean and well-maintained databases ensure high productivity of employees who can take advantage of that information in a broad range of areas, from customer acquisition to resource planning. Businesses that actively improve their data consistency and accuracy also improve their response rate and boost revenue.
Cleaning your data is a must
Businesses that take proper care of their databases are rewarded with these and many more benefits. Organizations that keep business-critical information at a high-quality gain a significant competitive advantage in their markets because they’re able to adjust their operations to the changing circumstances quickly.
At Sunscrapers, clean data is the starting point for any successful data science project, especially for building sophisticated solutions like machine learning algorithms. We always take proper care to clean data and make sure that our projects bring maximum benefits to our clients and their data management practices.
Are you looking for more information about data cleaning and more data-related topics? Follow our company blog, where our experts share their knowledge about data science with our community, or contact us at [email protected].