Przemek Lewandowski's articles
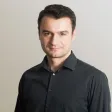
Przemek Lewandowski
Co-founder & CTO
Przemek is the co-founder and CTO of Sunscrapers. After graduating from the Warsaw University of Technology, he worked as a software consultant. At Sunscrapers, Przemek acts as the technical leader who supervises high-quality service delivery, helps to solve problems, and mentors other team members. Przemek is a passionate community activist, he leads open-source projects, volunteers in projects like Django Girls London, and organizes/speaks at tech events like PyWaw.
Search
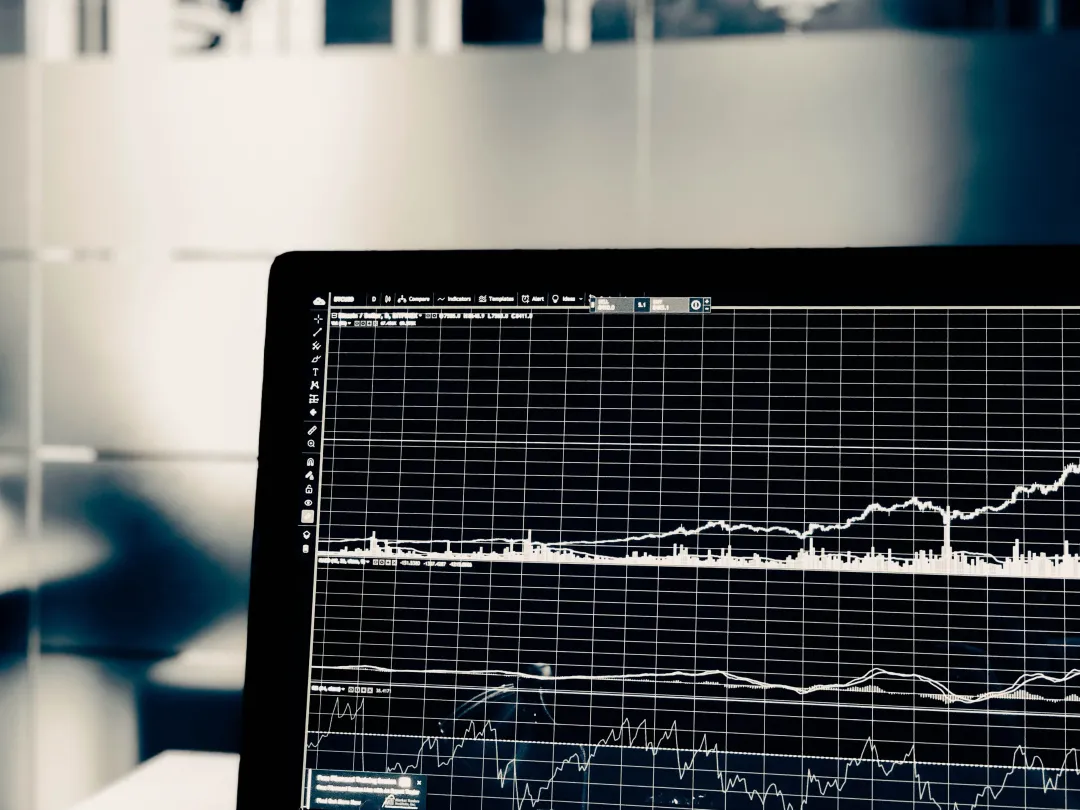
What is data cleaning and why is it important?
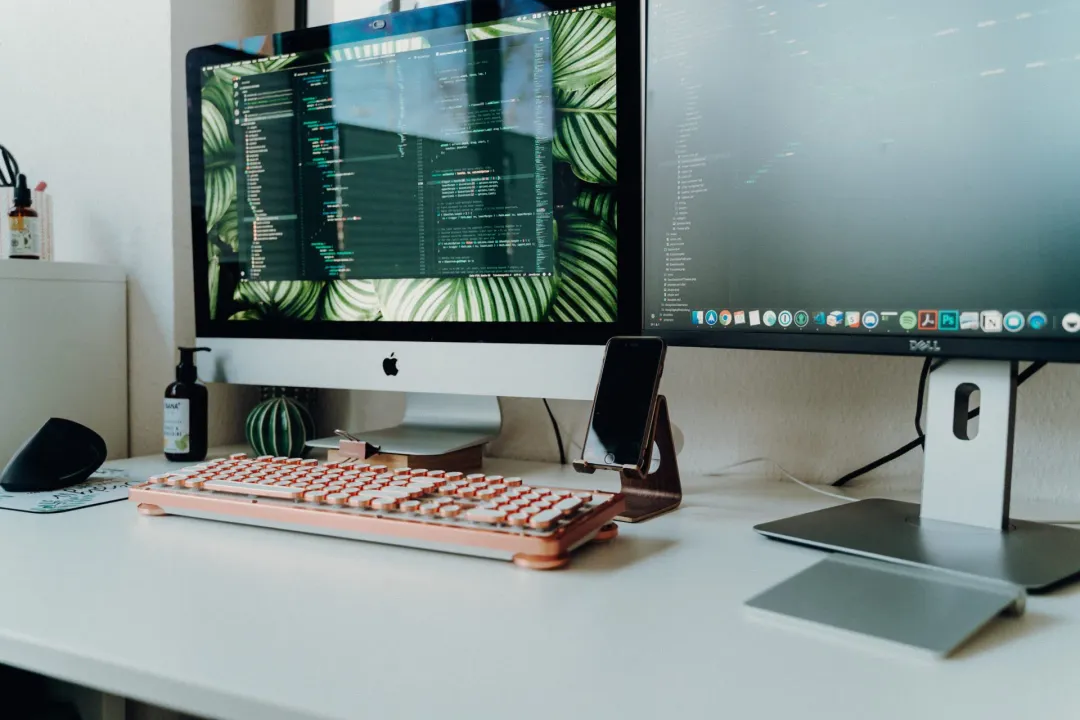
14 Python resources for intermediate and advanced Python developers
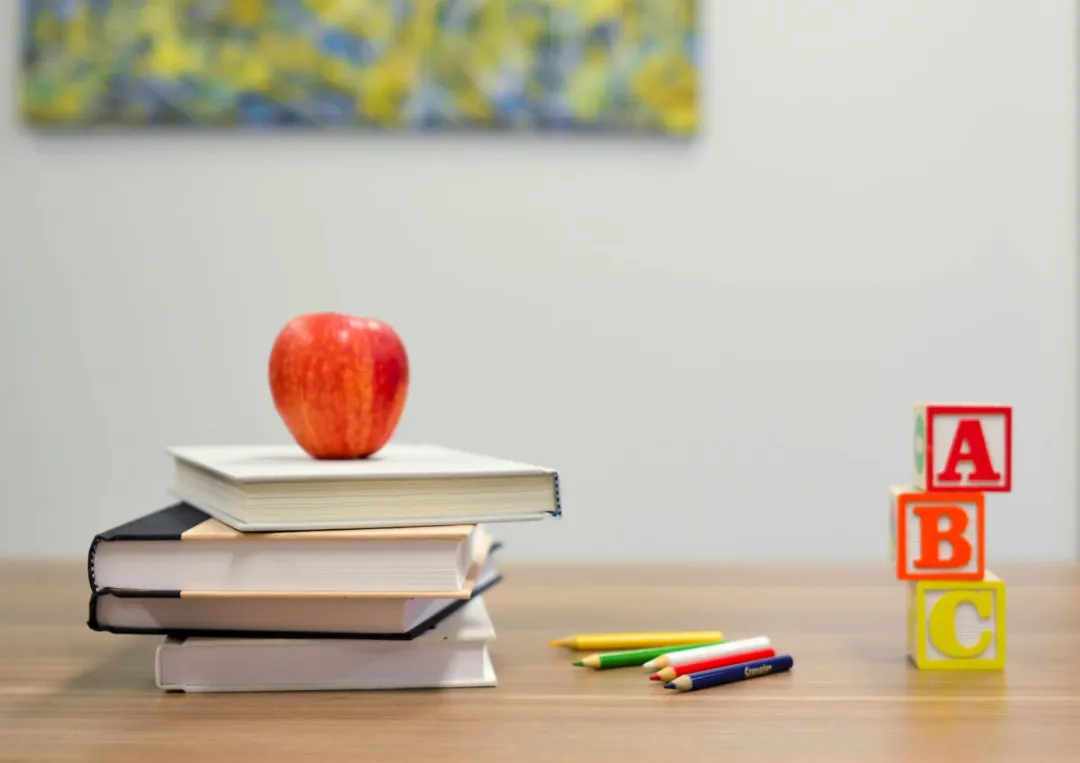
12 Best Python Tutorials For Beginners
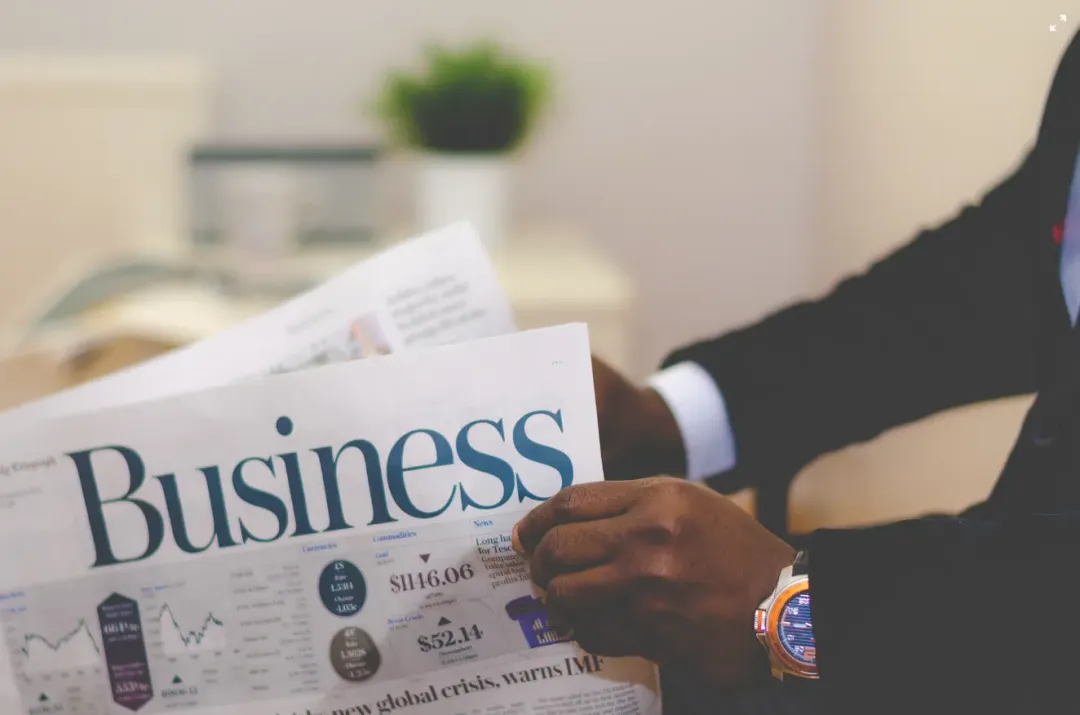
4 business challenges Machine Learning solves
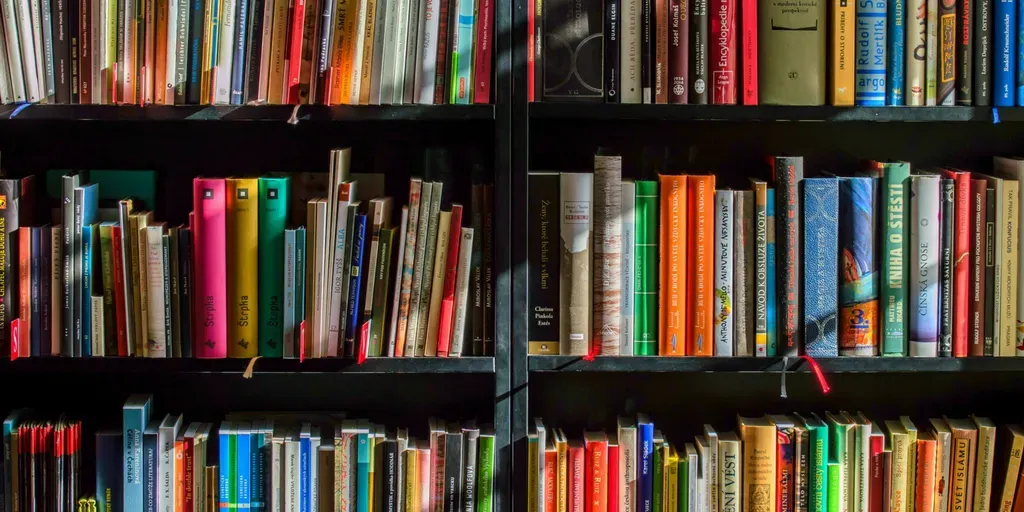
8 resources to help you become a better developer
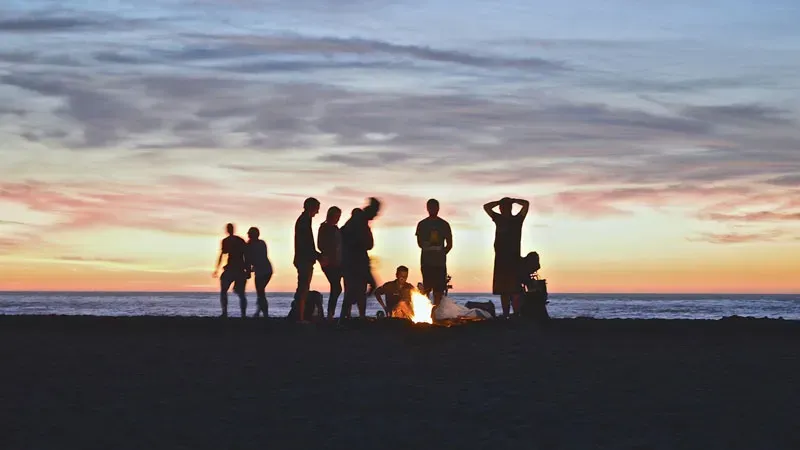
How to build great development teams
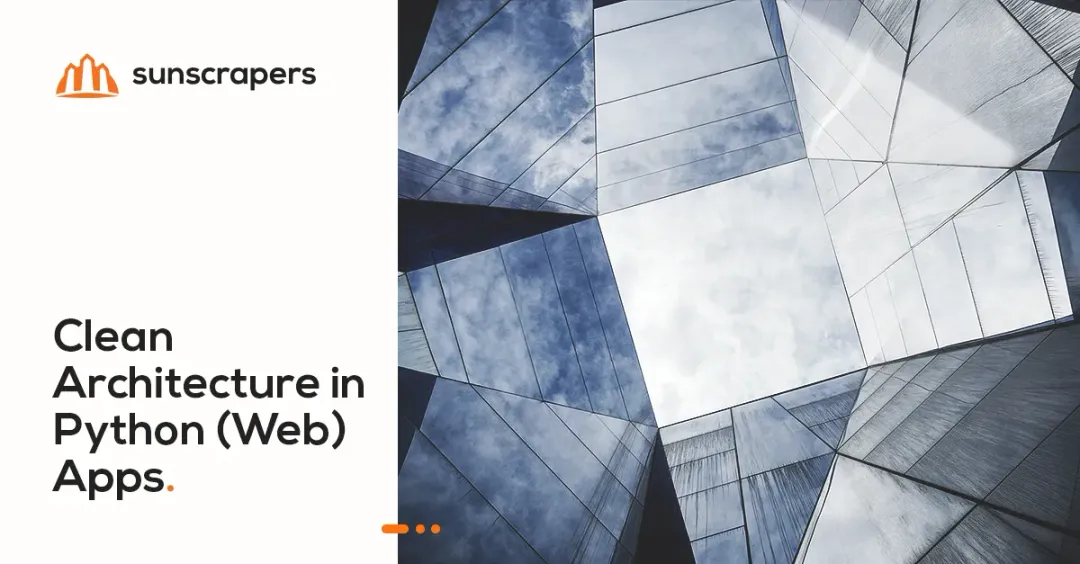
Short guide to clean architecture in Python web apps
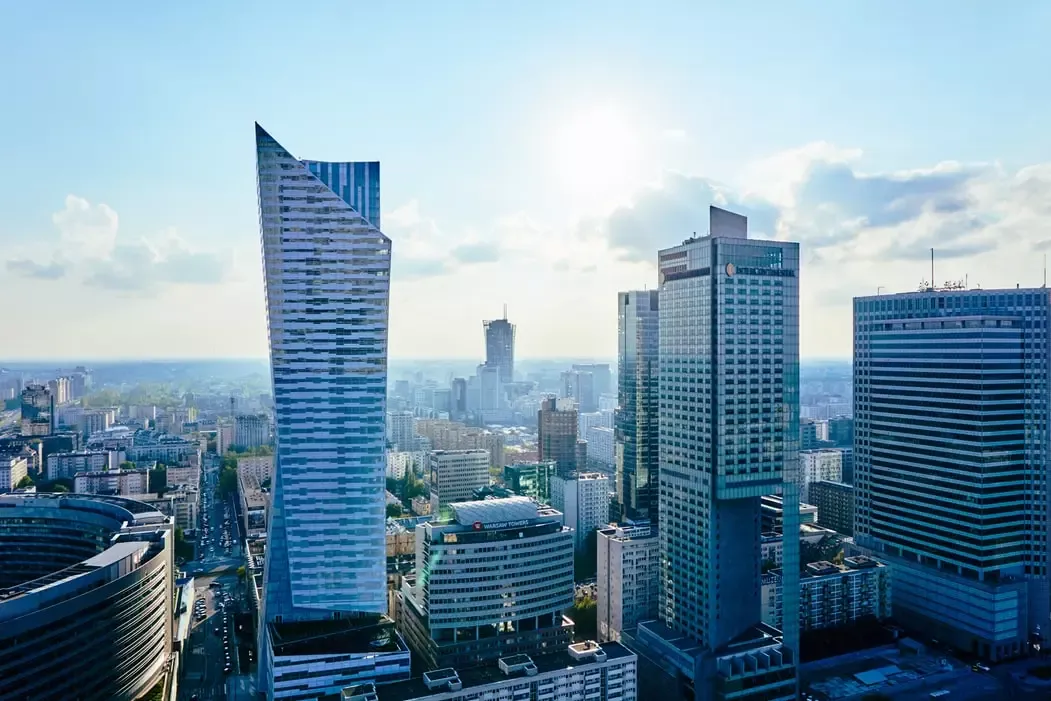
How to pick the right programming language (and why it should be Python)
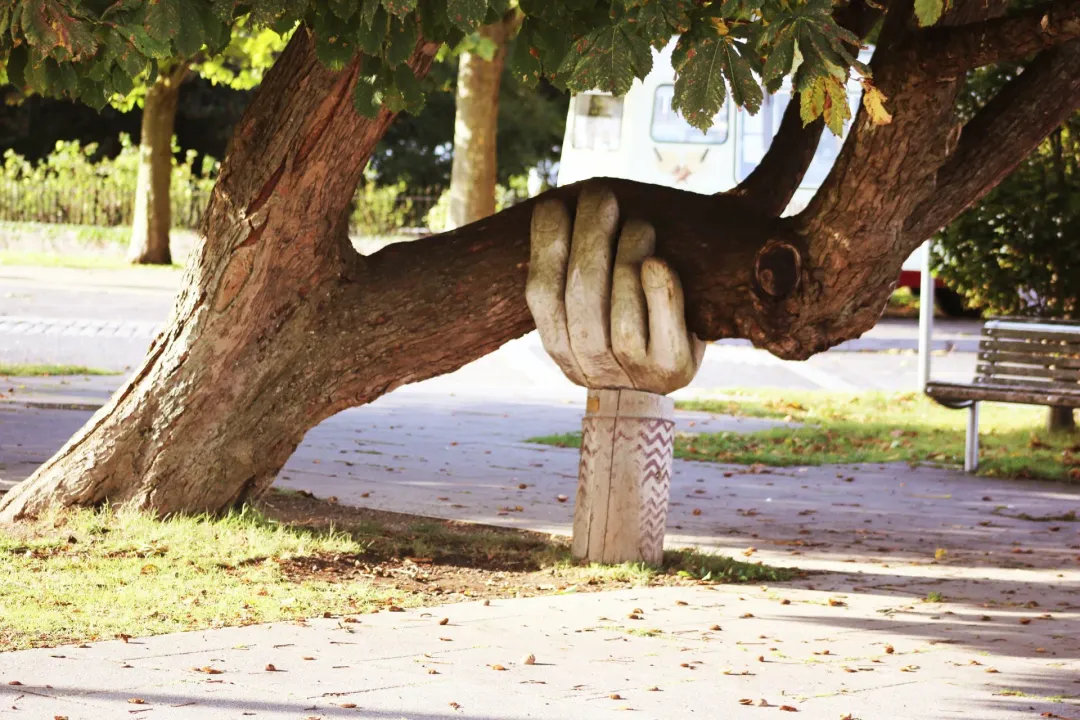