
Przemek Lewandowski
3 July 2018, 5 min read
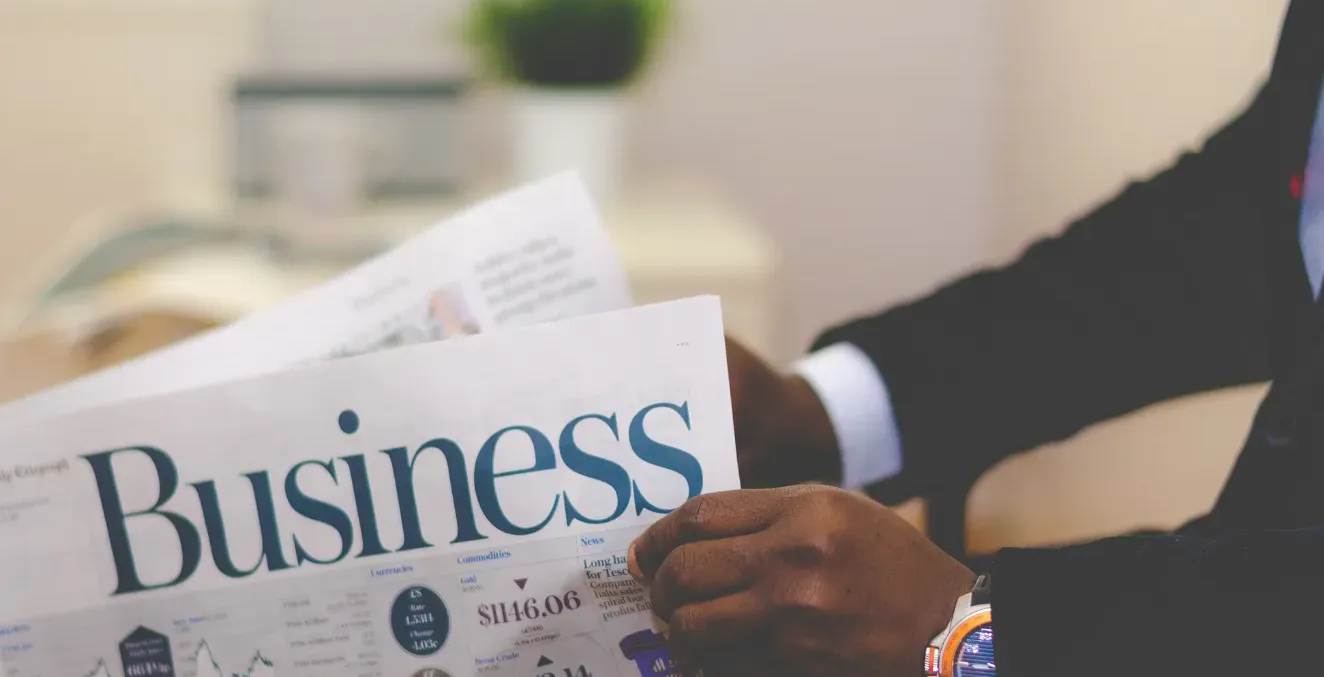
What's inside
- Problems that require analytics of massive data
- Problems that are affected by the human bias
- Problems that require precision
- Problems that are too expensive to be solved by humans
- When machine learning comes in handy
During the last few years, machine learning became a key industry buzzword and practically the Holy Grail people believe would solve every kind of business problem. Let's get one thing clear: machine learning isn't going to answer all of your business problems.
However, the models and algorithms that are part of machine learning can be applied very successfully to address specific issues. In fact, sometimes machine learning is the best solution to a given problem.
When deciding to invest in machine learning, the first step is making sure that your problem actually requires that type of expertise. But how do you know which problems can be tackled with machine learning technologies?
According to Harvard Business Review, organizations should start by distinguishing between automation problems and learning problems. “Machine learning can help automate your processes, but not all automation problems require learning.”
In general, you can apply automation to a problem without learning, if the problem in question is relatively simple. If you're dealing with a task that has a predefined sequence of steps that are currently executed by a human, you can quickly arrange for a machine to do it instead without having to employ any machine learning algorithms.
To help you decide whether your problem requires machine learning expertise, here is a short guide to top 4 business challenges we believe machine learning helps to solve best.
Problems that require analytics of massive data
A well-trained machine learning algorithm is able to make a decision way faster than it takes a human to process input, analyze it, and come up with the conclusion. Moreover, a human analyst can only interpret so many data points a day. Algorithms, on the other hand, can try their analysis, evaluate it, and then adapt it to come up with better results several times per second!
That type of analytics comes in handy in the finance industry, for example in credit card fraud detection. Since criminals are coming up with new fraud methods all the time, systems need to quickly adapt in order to detect these patterns in real time and understand the common signals that point to fraud.
The same holds true for product recommendations. Every consumer has different preferences which additionally change over time. Amazon and Netflix use engagement and ratings that make up huge data volumes to predict what a given user might want to watch or buy next. That type of analytics is simply way too complex and time-consuming for human brains.
Problems that are affected by the human bias
Algorithms don't approach data sets in the same way as their makers. In fact, they continuously iterate their decision-making process and refine the way in which they work on data sets. Moreover, machine learning algorithms will try approaches humans never would, just because they know the world while the machine is learning from scratch.
Naturally, since humans create algorithms, these algorithms might – and in fact do – carry some of our biases. However, in situations where you need a data set to be analyzed as objectively as possible, it's smarter to rely on the machine rather than a human.
For example, machine learning algorithms will be able to pick up patterns in user behavior and determine which advertisements should be displayed to individual users on the basis of data about these behaviors, not some preconceived beliefs about who that type of user is and what they might like.
Problems that require precision
Even if they do their best, human analysts are only human, and they might suffer from inconsistencies in their work. They might not perceive the difference between 89% and 89,1%. The machine will see that difference and adjust its behavior every single time.
That type of precision is especially valuable in areas that rely on accuracy inaccessible to the human eye. Computer vision systems are a great example here. It's a breakthrough area in machine learning that allows medical professionals to take advantage of algorithms for image analysis and pick up information that serves diagnostic purposes.
Other experts also predict that machine learning will play an increasingly important role in diagnostic applications as it becomes more accessible - and medical professionals become more willing to share their data with algorithms.
Problems that are too expensive to be solved by humans
Developing and training a machine learning algorithm includes upfront costs, but it's an excellent decision in the long run. To put it simply, sometimes putting human analysts to a problem is too expensive. Smart organizations often give their algorithms tasks that are related to issues that aren't worth it for humans to solve.
For example, if an algorithm can pick up ways to optimize a process and help a team gain an extra 10% of improvement, that's a definite win. However, that same improvement isn't enough to justify the comparatively high cost of hours spent on the problem. Human employees need training, management, and all types of associated expenses like insurance, vacations, and salary. There is also the risk that they get sick or quit right in the middle of a key crunch.
An example of this is manual data entry. Some of the significant business problems arise from inaccuracy or duplication of data. Organizations that rely on data for their business operations tend to employ machine learning and predictive modeling algorithms that perform time-intensive data entry and documentation tasks. That way organizations can delegate higher value problem-solving tasks to their human workers.
When machine learning comes in handy
We hope this short list of problems that lend themselves to machine learning helps you in deciding whether your business can benefit from this technology. There's no denying that machine learning can bring in a lot of value to enterprises, but before bringing algorithms on board it's a good idea to make sure that you actually need their help – or could do with simple automation software.
Do you know any other problems that machine learning solves? Or perhaps you use machine learning algorithms successfully to solve your business problems?
Share your thoughts in comments and be sure to check our blog for more updates about the art and science of machine learning.