
Maria Chojnowska
9 August 2023, 11 min read
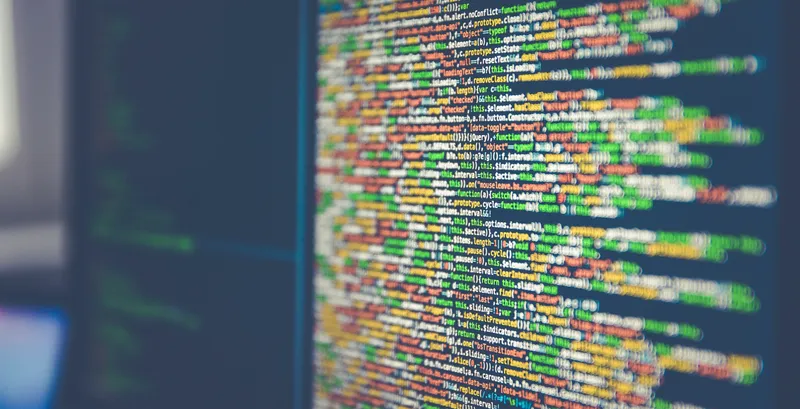
What's inside
- Crafting the Backbone of Data: How to Create a Data Catalog
- Methods of Data Cataloging: What Works Best?
- The Hallmarks of an Effective Data Catalog
- Tool Time: An Examination of Top Data Catalog Tools
- Emerging Trends: The Future of Data Cataloging
- Conclusion
In an era where data has become the lifeblood of organizations, it's no longer just about quantity but also about how you manage and leverage this crucial resource. Companies generate massive amounts of structured and unstructured data daily. Therefore, efficient cataloging, management, and utilization of this data become vital for driving business decisions and competitive advantage. This article delves into the best practices for building and maintaining a data catalog, explores the most potent tools, and discusses the latest industry trends.
The importance of a data catalog tool cannot be overstated. These tools act as the nerve center of an organization's data ecosystem, creating a centralized, user-friendly environment where metadata about the data is stored. They answer key questions: Where does the data come from? What does it mean? Who can access it? How does it relate to other data within the system? Navigating these seas of data becomes a breeze with an efficient data catalog tool at the helm.
Crafting the Backbone of Data: How to Create a Data Catalog
Creating a data catalog is an art and a science. While the process can vary depending on specific business needs, certain best practices hold universal appeal for advanced users.
-
Start by identifying different data types and sources within your organization. Don't get lost in the vastness of the data ocean; pinpoint the most relevant datasets.
-
Not all data catalog tools are created equal. Choose a tool that balances automation and manual cataloging capabilities with features for collaboration, robust security, and user-friendly search and navigation.
-
With the right tool in hand, begin the data entry process. This can involve a mix of automated and manual entries, combining the speed of AI with the precision of human understanding.
-
Data governance can make or break your data catalog. Define clear rules regarding data access, usage, and updates.
-
Data is not static, and neither should your catalog. Regularly update your catalog to accommodate new data and changes to existing datasets.
Actionable Steps to Build a Data Catalog
-
Conduct a Data Audit: Map out all existing data sources within your organization. Identify the data types, where they reside, their owners, and their importance to your operations.
-
Define Your Data Governance Policy: Before you start cataloging, set clear rules about data access, usage, and update. Establish who has the right to access and modify different types of data.
-
Choose Your Data Catalog Tool: Evaluate the different tools available on the market. Consider factors such as their cataloging capabilities (manual, automatic, hybrid), collaboration features, security measures, and user-friendliness. Don't forget to factor in your budget as well.
-
Prepare Your Data: Ensure your data is ready for cataloging. This could involve cleaning the data, addressing missing or inconsistent data, and possibly standardizing data formats.
-
Populate Your Catalog: Begin the cataloging process. Depending on your tool and resources, this could be fully automated, fully manual, or a combination of both.
-
Test and Refine: Once your catalog is set up, test it with a subset of users. Gather feedback and make necessary adjustments.
-
Roll Out: After refining, roll out the catalog to all relevant users.
-
Maintain and Update: Regularly update the catalog to incorporate new data, reflect changes in existing data, and enhance functionalities based on user feedback. This is a continuous process, not a one-time event.
By following these steps, you'll have a well-structured, efficient, and effective data catalog that serves your organization's needs. Remember, building a data catalog is a strategic process that may require time and resources. Still, the payoff in improved data accessibility, governance, and decision-making capabilities is well worth it.
Methods of Data Cataloging: What Works Best?
Manual cataloging, automated cataloging, or a mix of both? It's the question that all data management professionals grapple with.
-
Manual Cataloging: A method that, while time-consuming, allows for precise and context-specific cataloging, invaluable in complex or nuanced data landscapes.
-
Automated Cataloging: The efficiency champion. AI and machine learning tools expedite cataloging, making it ideal for handling vast data.
-
Hybrid Cataloging: The best of both worlds. This method combines the detailed insight of manual cataloging with the efficiency of automation.
The right approach?
The right approach for data cataloging depends on several key factors related to the managed data's complexity, volume, and unique characteristics. Let's delve deeper into each of these factors:
- Complexity of the data.
The complexity of the data refers to its structure, format, and inherent intricacies. Some data sets are straightforward and have well-defined schemas, making them suitable for automated cataloging. Automated cataloging tools can efficiently extract metadata and apply predefined rules to categorize and organize such data. However, manual cataloging becomes essential if the data is unstructured, inconsistent, or lacks clear patterns. Human expertise is needed to understand the nuances, apply context-specific knowledge, and accurately categorize the data.
- The volume of the data
The managed data volume plays a significant role in determining the cataloging approach. Manual cataloging can be time-consuming and impractical when dealing with large volumes of data. In such cases, automated cataloging offers efficiency advantages by rapidly processing and categorizing massive amounts of data. On the other hand, for smaller data sets, manual cataloging can provide detailed insights, ensuring precision and accuracy. It allows for a more thorough understanding of the data and enables context-specific cataloging.
- Unique data characteristics:
Each dataset possesses unique characteristics that impact the cataloging approach. Some data may require deep domain expertise or specialized knowledge for accurate categorization. For example, manual cataloging allows experts to interpret and classify the data correctly in scientific research or medical fields, where data may contain complex terminologies or domain-specific concepts. Additionally, certain data types, such as multimedia or unstructured text, may require advanced techniques like natural language processing or image recognition, which automated cataloging tools can facilitate.
It's essential to strike a balance between accuracy, efficiency, scalability, and contextual understanding. In some cases, a hybrid cataloging approach combining manual and automated methods may be the best solution. This approach leverages the strengths of both approaches, utilizing automation for efficiency and scalability while relying on human judgment for accurate interpretation and nuanced categorization.
The Hallmarks of an Effective Data Catalog
Just as a great novel isn't merely a collection of words, a good data catalog is not just about having a repository for metadata. An effective data catalog for advanced users should be:
-
Comprehensive and Accurate: It should provide complete, up-to-date metadata for every dataset.
-
Collaborative: Users should be able to comment, rate, or provide feedback on datasets, fostering a community of data users.
-
Transparent: Every data's origin, journey, and lineage should be clearly stated.
-
Secure: Strict controls should be in place to restrict access based on user roles and responsibilities.
-
Integrative: The catalog should work seamlessly with other data management and analysis tools.
Tool Time: An Examination of Top Data Catalog Tools
There's a wealth of data catalog tools on the market, but here we spotlight three power players:
-
Alation: Known for its prowess in automated data discovery, indexing, and cataloging, Alation offers a potent blend of data governance features and collaboration tools.
-
Collibra: This tool shines in the realm of data governance, offering a user-friendly interface and comprehensive metadata management.
-
IBM Watson Knowledge Catalog: A tool that harnesses the power of IBM's advanced AI. Watson offers automated data cataloging coupled with robust security and governance features.
Detailed Review of Top Data Catalog Tools
Feature | Pros | Cons |
---|---|---|
Automated Data Discovery | Automated scans and indexes data sources. | Quick and efficient data cataloging thanks to automation. |
May require significant initial configuration and tuning. | ||
Machine Learning Suggestions | Provides smart suggestions to users for data discovery. | Strong collaborative features encourage data democratization and team synergy. |
Could be expensive for smaller businesses. | ||
Collaboration Tools | Enables users to discuss data, share knowledge, and maintain a common understanding. |
Feature | Pros | Cons |
---|---|---|
Comprehensive Metadata Management | Maintains detailed information about each dataset. | Great for organizations needing strong data governance and compliance features. |
Automation features may not be as strong as some competitors. | ||
Data Governance Center | Provides strong governance features for compliance and control. | Users appreciate its intuitive interface at all levels of technical proficiency. |
The cost can be prohibitive for smaller organizations. | ||
User-friendly Interface | Designed to be easily navigated by non-technical users. |
Feature | Pros | Cons |
---|---|---|
AI-powered Data Cataloging | Utilizes Watson's AI capabilities for automated cataloging. | Takes advantage of IBM's advanced AI for efficient cataloging. |
Can be complex to set up and use, requiring technical expertise. | ||
Robust Security and Governance | Enforces policies for data privacy and security. | Strong security and governance tools make it a good choice for organizations with stringent data security needs. |
Cost can be a concern for smaller businesses, typical of many IBM products. | ||
Integrative Capabilities | Works well with other IBM data management and analysis tools. |
Each tool has unique strengths, and your organization's specific needs should dictate the choice between them, and constraints should dictate the choice between them. It's also important to note that these are just three of many data catalog tools available on the market. Always consider multiple options and conduct a thorough analysis before making a decision.
Emerging Trends: The Future of Data Cataloging
In the fast-paced world of data management, staying on top of trends is essential.
-
AI and Machine Learning: The rise of automated data cataloging, thanks to advances in AI and machine learning, is a trend too powerful to ignore.
-
Data Privacy and Governance: As regulatory requirements multiply, data catalogs' role in ensuring compliance is becoming even more critical.
-
Unstructured Data Cataloging: The growth of big data necessitates the development of effective methods for cataloging unstructured data like text documents or social media posts.
-
Cloud Integration: As businesses migrate to the cloud, data catalogs that can handle cloud data are in high demand.
Conclusion
In conclusion, choosing the right cataloging approach is a critical decision for data management professionals. It requires a deep understanding of the complexity, volume, and unique characteristics of the data at hand. While manual cataloging offers precise and context-specific categorization, it can be time-consuming and impractical for large datasets. On the other hand, automated cataloging tools expedite the process, making them ideal for handling vast amounts of data. Still, they may lack the nuanced understanding required for complex or domain-specific data.
Finding the optimal approach often involves striking a balance between efficiency, accuracy, scalability, and contextual understanding. The hybrid cataloging approach, combining manual and automated methods, emerges as an attractive solution, leveraging the strengths of both approaches. It empowers organizations to benefit from automation's efficiency while harnessing human expertise for accurate interpretation and nuanced categorization.
However, the journey of data cataloging does not end with the initial approach. It requires continuous evaluation and adaptation as the data landscape evolves. New data sources, changing requirements, and emerging technologies will continue to shape the cataloging strategies of the future.
Ultimately, data cataloging aims to unlock the full potential of data assets, enabling organizations to make informed decisions, extract meaningful insights, and drive innovation. By understanding the complexities of their data and selecting the appropriate cataloging approach, data management professionals can lay a solid foundation for effective data governance, compliance, and utilization.
As the realm of data expands, organizations embracing a thoughtful and adaptable cataloging approach will be better positioned to thrive in the data-driven era. By leveraging the power of technology and human expertise, they can unlock the actual value of their data and harness its potential to drive transformative outcomes.