
Maria Chojnowska
21 December 2023, 9 min read
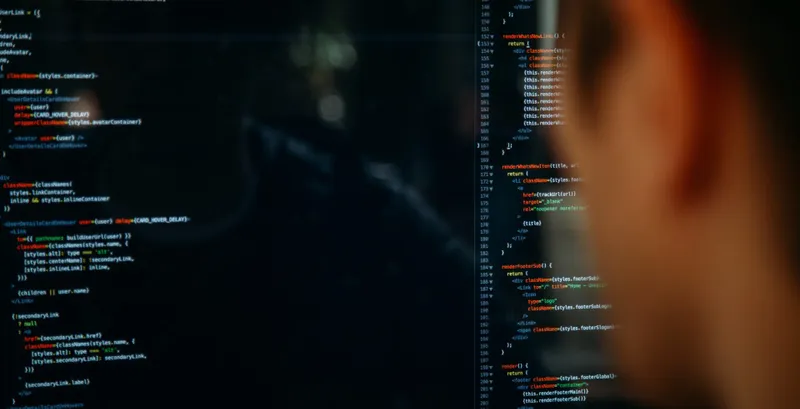
What's inside
- What is Machine Learning?
- Examples of Machine Learning
- What is Data Mining?
- Examples of Data Mining
- Distinguishing Data Mining and Machine Learning
- Conclusion
Here's something practically every company out there is focusing on today: data. Organizations are collecting and processing data in unprecedented volume. They’re also using sophisticated data analytics solutions that unlock valuable business insights.
We have supported companies of different sizes on their digital transformation journey by providing them with tailored data science services. Our experience shows that "data science" and related terms such as "machine learning" or "data mining" can generate a fair amount of confusion.
Read on to discover the key similarities and differences between data mining, machine learning, and data science.
What is Machine Learning?
There are many machine learning definitions out there, but here's a simple one. Machine learning is a **subfield of data science that deals with algorithms able to learn from data and make accurate predictions-**innovative approaches such as neural networks and deep learning. Machine learning uses supervised and unsupervised learning methods to train algorithms.
Examples of Machine Learning
To help you understand the capabilities of machine learning, here are three applications of this technology that power many real-world products.
Image Recognition
This is one of the most common use cases of machine learning which is gaining traction across many different industries. The idea here is building software that can identify and classify objects found on digital images or videos. For example, developers can use machine learning to build a tool that recognizes human faces in images and then matches them to pictures stored in a database. Image recognition software has found many applications in sectors such as retail, healthcare, and entertainment. For instance, it enabled the healthcare industry to introduce new microsurgical procedures delivered by robots that use computer vision and image recognition techniques.
Speech Recognition
Another common use case of machine learning is speech recognition applications. Such solutions can recognize spoken language and convert it into a text file or take action if following voice commands. Virtual assistants such as Amazon’s Alexa or Google Now rely on speech recognition to bring their users a rich selection of possible actions they can take. Such voice user interfaces allow performing voice searches, dialing, appliance control, call routing, or even data entry.
Medical Diagnosis
The powerful capacities of machine learning are transforming healthcare. The technology currently finds many uses in disease diagnostics. Doctors use it for analyzing clinical parameters and their specific combinations for predicting disease progression, therapy planning, and patient monitoring. Healthcare providers also use AI-powered chatbots equipped with speech recognition capabilities to identify patterns in patient symptoms and help generate a potential diagnosis or even recommend an appropriate course of action.
What is Data Mining?
Data mining is an interdisciplinary field that concentrates on discovering the properties of data sets. To accomplish that goal, data mining can take on different approaches. Machine learning is one of them. Other approaches include topological data analysis or data visualization.
Examples of Data Mining
Here are three real-world use cases of data mining demonstrating this approach's versatility to analyze data.
Marketing
Enterprises use data mining to explore their increasingly large databases of customer data. By analyzing the relationships between customer demographic data and other parameters, such solutions can predict their behavior to direct personalized marketing campaigns. Such tools also come in handy for market segmentation that requires processing large volumes of data. Data mining helps companies to understand customer interests and preferences to deliver more effective marketing messages and products.
Retail
Stores and supermarkets use data mining to generate joint purchasing patterns that identify product associations. Such insights help stores decide how to place items in the aisles and on the shelves to generate maximum revenue. Data mining also helps to identify which offers customers value most and increase sales by delivering such offers to the right people at the right time – for example, at the checkout queue.
Banking
Banks use data mining tools for a broad range of purposes. Market risk is one prominent area of application. Financial services companies apply data mining to credit ratings. The technology also powers anti-fraud systems that analyze transactions, purchasing patterns, card transactions, and customer financial data to identify threats. Banks can learn more about their customers by gathering data about their online behaviors. Data mining helps to optimize the ROI of marketing campaigns, manage regulatory compliance obligations, and learn more about the performance of individual marketing channels.
Distinguishing Data Mining and Machine Learning
Now that you know what machine learning and data mining are, you're probably still asking yourself: What's the difference between machine learning and data science? Is there anything the two have in common? And where does data mining enter the equation?
It's worth taking a moment to answer these questions. That's how you deepen your understanding of data science and analytics and the potential benefits they might bring to your company.
Let's start with this:
Data mining and machine learning are both rooted in data science.
However, there are several key distinctions between these two areas. We list a few of them below.
Learning Source
a) Data Mining
At its core, data mining is an analytical process. It's about delving deep into vast datasets and seeking patterns, correlations, and anomalies. Imagine a vast ocean of data: data mining is casting a net to gather insights, much like a fisherman seeking a catch. A practical example is a fashion company sifting through years of sales records, customer feedback, and market trends. They're not just looking for what sold well—they're searching for patterns to understand why certain products resonated with consumers and predict the next big trend.
b) Machine Learning
Machine learning takes the concept a step further. Instead of merely fishing for insights, machine learning teaches the net to become smarter with each cast. It's not just about identifying patterns—it's about understanding and learning from them, enabling predictive and even prescriptive analytics. For instance, consider a streaming platform like Netflix. With machine learning, it doesn't just recognize that a user watches a lot of sci-fi—it understands the nuances of their preferences. It recommends shows they will enjoy, refining its suggestions over time.
Pattern Recognition
a) Data Mining
If data is a puzzle, data mining is putting those pieces together meaningfully. It harnesses algorithms, statistical techniques, and more to uncover hidden patterns and relationships within unstructured data. For instance, e-commerce platforms might use data mining to determine which products frequently get bought together, enabling better product bundling.
b) Machine Learning
While machine learning involves pattern recognition, it adds a layer of adaptability. It doesn't just recognize patterns—it anticipates and adjusts to them. For example, cybersecurity systems powered by machine learning don't just detect known malware. They analyze behaviors and adapt to recognize new, previously unseen threats.
Applications
a) Data Mining
This technique has a wide array of applications, depending on the industry. Data mining might help discover correlations between market trends in finance, enabling better investment strategies. For marketers, it can reveal insights about customer behavior, optimizing advertising campaigns. Data mining offers a deep dive into existing data to extract actionable insights.
b) Machine Learning
The applications of machine learning are vast and growing. It's the engine behind many modern conveniences and innovations. Ride-sharing apps like Uber use it to predict ETAs. E-commerce giants like Amazon harness it for real-time product recommendations. Machine learning can analyze patient data in healthcare to predict disease outbreaks or recommend personalized treatment plans. What sets machine learning apart is its ability to continually refine its processes and improve over time, offering increasingly accurate predictions and solutions.
Data Mining | Machine Learning | |
---|---|---|
Learning Source | Data mining is casting a net into vast datasets to gather insights, like a fisherman seeking a catch. | Machine learning teaches the net to become smarter with each cast, refining its understanding based on patterns. |
Pattern Recognition | Data mining combines the puzzle pieces of data to uncover hidden patterns using algorithms and statistical techniques. | Machine learning anticipates and adjusts to recognized patterns, adapting to new and unseen data behaviors. |
Applications | Data mining dives deep into existing data across industries to extract actionable insights. | Machine learning refines its processes over time, powering modern innovations and offering increasingly accurate predictions. |
Conclusion
Let's circle back to the fundamental questions we set out to address:
- What are Data Mining and Machine Learning?
Data Mining is about delving deep into vast datasets to discover patterns, correlations, and anomalies. At the same time, Machine Learning is a subfield of data science focusing on algorithms that can learn from data to make accurate predictions.
- How are they applied in real-world scenarios?
Machine Learning powers innovations like image recognition, speech recognition, and medical diagnosis. In contrast, Data Mining is essential for marketing insights, retail strategies, and banking applications.
- What differentiates Data Mining from Machine Learning?
While both are rooted in data science, Data Mining is analogous to casting a net into data to find insights. In contrast, Machine Learning refines its processes based on patterns it identifies, becoming smarter with each iteration.
- How can businesses choose the right technology for their needs?
Understanding the unique strengths and applications of both is crucial. While there's an overlap, businesses should opt for Data Mining for deep dives into existing data and Machine Learning when adaptability and predictive capabilities are prioritized.
Data Mining and Machine Learning offer invaluable tools for deriving insights from vast data sets. Choosing the right tool becomes imperative as businesses tread the path of digital transformation. Understanding their distinct roles can illuminate the way forward for your data endeavors, whether it's the depth of Data Mining or the adaptability of Machine Learning.
Amidst our discussion, one pivotal question stands out that needs further emphasis:
What distinguishes "data science" from "machine learning"?
"Data science" is a broad field encompassing many techniques and methods for data analysis, including statistics, data processing, data visualization, and many others. In contrast, "machine learning" is a subset of "data science", primarily focusing on algorithms and models that can learn from and make decisions or predictions based on data. Understanding this distinction is crucial to appropriately harnessing the potential of each in specific business scenarios.
Need expert insights on choosing the right data solution for your business? We're here to guide you through the ever-evolving landscape of data science technologies. Contact Sunscrapers today and help us illuminate the path forward for your data endeavors.