
Sunscrapers Team
13 January 2020, 9 min read
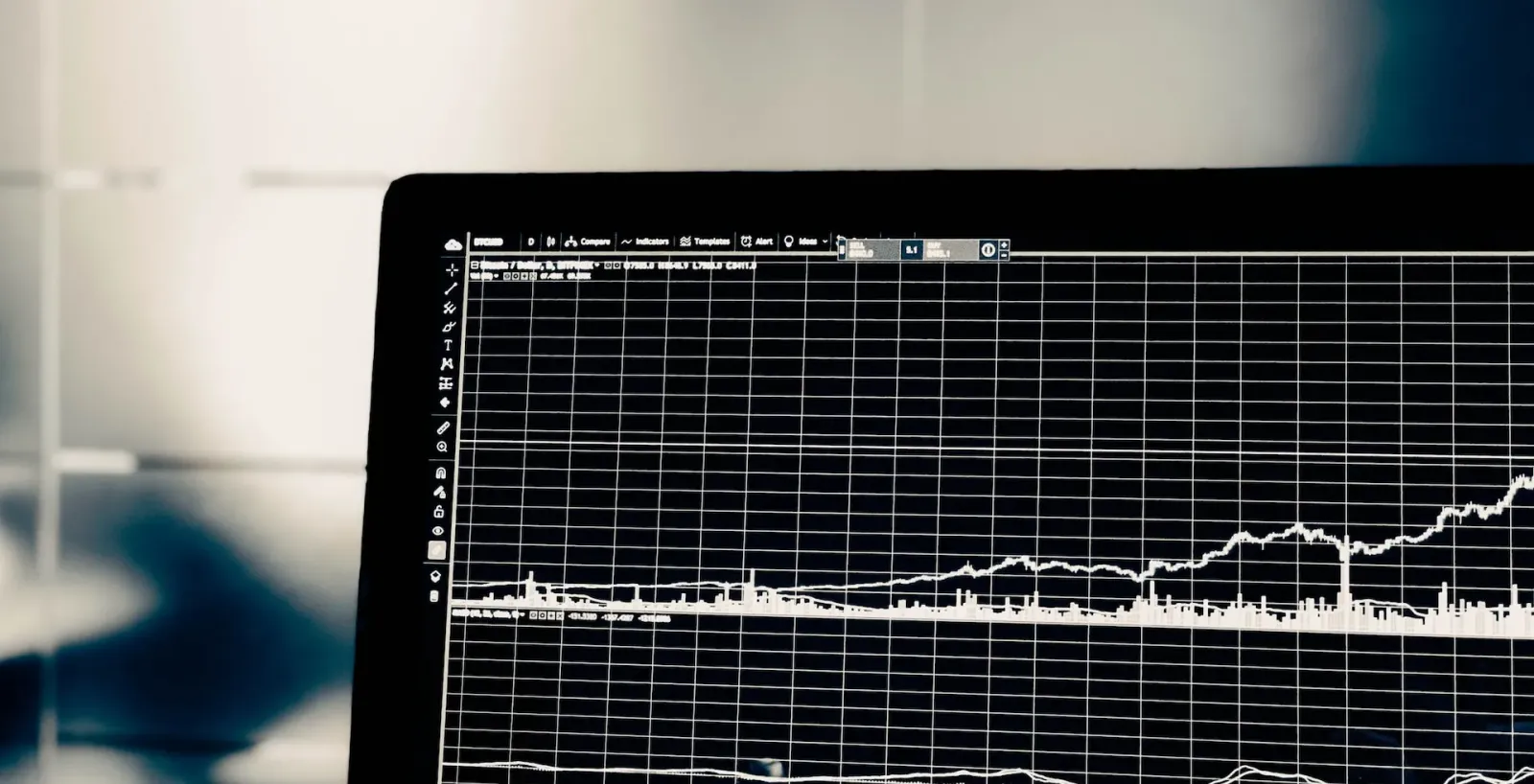
What's inside
- Machine learning on the enterprise landscape
- Top machine learning use cases
- How to get started with ML in the enterprise
- It all comes down to picking the right problem
- Conclusions
- Resources
Artificial intelligence (AI) and its most important subset, machine learning and deep learning, are causing a profound disruption across many different industries. Marketing specialists take advantage of AI to deliver personalized messages to customers, automotive companies build smart car technologies, and physicians employ AI solutions for diagnostic purposes.
Before we knew it, AI solutions have become the cornerstone of the digital economy. And the impact of this technology on companies will become even greater in the future. According to IDC, global spending on AI will reach $57.6 billion by 2021, seeing major investments from sectors such as banking, healthcare, and retail.
Machine learning is rapidly transforming enterprise technology. It marks the next chapter in the digital transformation journey enterprises have embarked on during the last decade. Today, it's the enterprise innovation labs that contribute to shaping the future of applied ML.
Successful enterprise adoption of ML solutions requires different teams to come together and align technical capabilities with real-world business problems. To make the most of this technology for your business, you need to understand what its unique capabilities are and what are some frameworks you can use to start your journey.
Read on to find out the most common ML use cases in companies and how to take the first steps towards your own implementation.
Machine learning on the enterprise landscape
Before delving into the details, here's a broader look at the enterprise ML use cases on the market.
A recent survey revealed that one of the critical areas the technology is expected to revolutionize is customer interactions. The most popular applications here are solutions that generate customer insights and intelligence, interact with customers, improve the customer experience, retain customers, and increase customer satisfaction. The largest companies expect AI technologies to help them increase customer loyalty.
In general, larger companies and sophisticated adopters of innovative technologies are investing more in implementing machine learning and deep learning across a wide range of applications. As they invest resources into ML, they learn where to apply it for best results.
There's also an interest among these companies in training AI for reducing costs. In the survey, 44% of them mentioned cost reduction as one of their primary use cases. Early-stage adopters mainly focus on improving customer retention – that's what 60% of them stated. Middle-stage adopters of ML are split between increasing customer loyalty (38%) and reducing costs (39%).
What about the particular problems companies are solving with ML? This report on the basis of a survey of over 600 executives listed the top six use cases for AI and ML in organizations:
- Data security (28%) – including early threat detection, risk identification, improving operation, and corrective action.
- Real-time analytics (24%) for identifying product offers, fraudulent transactions, dynamic pricing, and other.
- Personalized data visualizations and dashboards (24%) – such dashboards help support predictive analytics and indicate potential performance improvements.
- Data integration, preparation, and management (23%) - often in combination with cloud computing.
- Sales/revenue forecasting (23%).
- Personal security (20%) – with applications such as home surveillance or in financial services security.
Top machine learning use cases
The hype surrounding AI and ML has made it very difficult for companies to separate futuristic applications from real-world opportunities. To make sense of the potential value this technology can bring to a company, CIOs and other decision-makers need to get familiar with practical use cases of machine learning. Here are top ML use cases for enterprise applications.
1. Process Automation
There's no denying that one of the key AI use cases is the intelligent process automation. By combining artificial intelligence technologies and automation, companies can automate tasks ranging from simple ones (like manual data entry) to more complex ones (like life insurance risk assessment or other financial services).
ML comes in handy for scenarios where the human decision-making process is essential but set within specific constraints or patterns. Natural language processing, machine vision, and deep learning are technologies that boost traditional rule-based automation and learn how to perform tasks better as they adapt to change.
Process automation tools help businesses to reduce operational costs, use their resources better, make decisions faster, and boost operational efficiency. By freeing human workers to focus on what matters, ML and deep learning applications allow companies to build more innovative products and services.
2. Security
Another area where the technology is helping enterprises improve their operations is security. In particular, ML helps in analyzing threats and responding to security incidents. According to ABI research, machine learning and data security will increase spending $96 billion by 2021.
ML powers predictive analytics tools that enable early detection of threats. Any anomalies within the system will never go unnoticed thanks to solutions that learn to become more effective with every exposure to data. Organizations that invest in such tools are able to navigate regulatory requirements and become innovation-driving leaders in their sectors.
3. Sales and marketing optimization
Sales and marketing departments generate a lot of data companies can use to improve their strategies and generate more leads. To unlock the value of this data, they need ML solutions. Such tools can perform functions such as predictive lead scoring, content personalization, intelligent content, customer sentiment analysis, churn predictions, and sales forecasting analysis. When synchronized together, they give enterprises a significant competitive advantage, regardless of their industry.
4. Customer service
Many organizations are investing in solutions that help to free up their human resources and automate as many customer service tasks as possible. Virtual assistants and chatbots are becoming a new standard in the world of customer service.
The volume of customer interactions organizations deal with is usually massive and generates a lot of data. Software data scientists can use it as training material for fine-tuning algorithms and building better learning models. By leaving routine customer service tasks to machines, enterprises can free up human employees to focus on more complex problems, be creative, and drive innovation, improving the speed of decision-making. According to Juniper Research, conversational assistants will generate $8 billion in cost savings annually by 2022.
5. Team collaboration
Here's another area where machine learning has a lot of potential for companies: team collaboration tools. Such tools improve the efficiency of human employees, accelerate the discovery of new ideas, and improve the results of teams that work from different locations.
Many businesses are planning to implement artificial intelligence in their unified collaboration and communication applications. These include video, audio, and image intelligence that can add context to the content teams share. Some companies are busy integrating a chatbot into their team application to enable functionalities like alerting team members or asking them for status.
How to get started with ML in the enterprise
Now that you know what kind of capabilities machine learning solutions can bring into your organization, you're probably wondering how to get started.
The web is full of fantastic resources on the topic. "How to spot a machine learning opportunity, even if you aren't a data scientist" from the Harvard Business Review is a good start.
We decided that it is worth sharing the experience our ML experts have gained while advising enterprise clients about implementing the technology. Here's our take on getting started with machine learning for organizations.
It all comes down to picking the right problem
Contrary to popular belief, ML isn't a universal antidote to them to all problems. Since implementing new technologies isn't cheap, it's a good idea to concentrate on a problem or area that may bring you a strong ROI.
Here are some critical steps you need to take to make sure that your ML or deep learning implementation brings you the best results:
- Start small – even if you'd like to this technology to address a significant problem at your organization, it's a good idea to scale it down. But you need to balance its size so that people still care and see the potential benefits ML may bring them. Otherwise, the project might not get the attention it needs, and its risk of failure will increase – or the risk that your leaders become disillusioned with ML.
- Have a measurable goal – make sure that your goal is clear and can be measured — machine learning algorithms to require a quantifiable outcome to optimize a task. For example, if your company is trying to predict whether a customer will buy one of your products, start with data that directly links that predictive data to outcome data – for example, the clickstream behavior to the sales transactions.
- Pick a problem of the right complexity – it seems that ML is the way to the future, but many solutions will work just fine with simple rules. ML makes that the biggest impact when the task is too complex to be encoded in rules. When picking the problem you'd like to solve with ML, make sure that it requires such complex technology.
- Data availability – the more historical data you have, the more resources your ML algorithm will have to learn from. As a result, it will become more accurate and successful. Check whether you're collecting the kind of information required to answer to address the problem. Alternatively, you partner with an experienced software development agency to get the data you need for your tool.
Conclusions
Enterprises looking to take advantage of machine learning and artificial intelligence will have to approach the problem from a broad perspective. First, they will need to answer a range of technical, data processing, and personnel questions.
They will need to gather a massive amount of quality data (often linked to a reliable cloud computing solution), make sure that employees are trained for the moment artificial intelligence is implemented, and choose the right technology partner experienced in ML.
Are you looking for expert data scientists to help you launch a new project? Get in touch with us; we help organizations like yours make the most from ML and AI.